Advanced Bayesian computation methods for estimation, prediction and control in multi-sensor complex systems (ADVENTURE)
We have recently witnessed the advent of new technologies that hold promise of great improvements to the well-being of elderly people and individuals who suffer from a number of health conditions. State-of-the-art sensor technology bundled together with light-weight computing and communication devices, for example, potentially enable the monitoring of patients within their homes, with a standard of care that just a few years ago was only possible at special units in hospitals.
Unfortunately, hardware technology alone is not enough to bring all that potential into reality. It guarantees fast and inexpensive access to a wealth of data —yet how to extract knowledge from it and how to make informed and useful decisions is a problem of a different nature. There is an exacting demand of models that impose structure on the data, in order to interpret it, and algorithms that combine those models and the data bunch to estimate key magnitudes, detect ongoing conditions or predict future events. In order to meet these demands, we advocate a Bayesian approach to statistical inference and learning, which encompasses the tasks of model design, comparison and validation, as well as the development of flexible algorithms that fully exploit the features and structure of the underlying models. Bayesian methods enjoy relevant advantages with respect to classical techniques. These include:
– generality, as they can be applied to broad classes of systems, possibly nonlinear and/or non-Gaussian,
– computational flexibility, when combined with Monte Carlo sampling and integration methods, quadrature and cubature techniques or variational schemes, and
– “complete” solutions in the form of probability distributions, which not only enable the computation of point estimates, but also error estimates, credibility intervals and other statistics.
Because of the typical scenarios encountered in health applications, we are primarily interested in the development of models and algorithms which can be implemented using arrays of light-weight wireless devices that incorporate sensing, communication and computing capabilities. This is, for example, the case of typical wireless sensor networks (WSNs) used for the monitoring of patients at their homes. We will investigate the specific issues of the implementation of the proposed Bayesian inference schemes on distributed systems, including parallelization, inter-node communication constraints, energy consumption or the optimization of the sensing and communication protocols. As a driving theme for this line of research, we will pursue new and efficient algorithms for indoor positioning using WSNs.
We aim at combining both computational and positioning enabling technologies to tackle three high prevalence problems in medicine:
– The functional evaluation of psychiatric patients (specifically, patients that suffer from schizophrenia, bipolar, and mood disorders).
– The quantitative characterization of epileptic seizures.
– The characterization and detection of rotors in atrial fibrillation (AF).
With the cooperation of a multidisciplinary research team, we will build three demonstration systems, using currently available hardware and software elements, to assess the effectiveness of the proposed solutions.

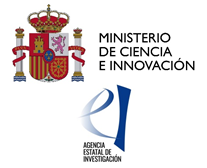